Businesses are constantly seeking ways to increase their output and increase their profits. Data mining’s predictive analytics field enables companies to do just that. Businesses can use predictive analytics technologies to make better decisions based on historical data and future-proof their operations. The top ten predictive analytics tools, broken down by category, will be covered in this blog post.
Predictive analytics tools can forecast future customer behavior, spot patterns, and catch fraud, among other things. The finest predictive analytics solutions will have a user-friendly interface, be able to handle enormous data volumes, and offer a variety of valuable capabilities.
RapidMiner Studio, SAS Enterprise Miner, Microsoft Azure Machine Learning Studio, and IBM SPSS Modeler are some of the best predictive analytics solutions available today. These effective predictive analytics techniques can boost a company’s bottom line.
Check out our list of the top 10 predictive analytics tools below if you’re searching for a tool to help you grow your business.

Top Ten Predictive Analytics Tools:
-IBM SPSS Modeler
A predictive analytics tool, IBM SPSS Modeler, can be used for several tasks, including trend detection, fraud detection, and forecasting future customer behavior.
-SAS Enterprise Miner
An effective predictive analytics tool with extensive capabilities and an intuitive user interface is SAS Enterprise Miner.
-Microsoft Azure Machine Learning Studio
Large data sets can be handled with the cloud-based predictive analytics application Microsoft Azure Machine Learning Studio.
-RapidMiner Studio
Another effective predictive analytics program with a wealth of capabilities and an easy-to-use interface is RapidMiner Studio.
-Alteryx Designer
A predictive analytics tool called Alteryx Designer makes it simple to evaluate data and build predictive models.
-Statistica
Data visualization and predictive modeling are only two of the many functions that Statistica’s predictive analytics software offers.
– KNIME Analytics Platform
Predictive analytics software called KNIME Analytics Platform is open-source, accessible, and suitable for some uses.
-H20 Driverless AI
H20 Driverless AI is a predictive analytics tool that uses artificial intelligence and has several functions, including autonomous machine learning.
-Minitab Statistical Software
Predictive analytics tools are part of Minitab’s complete statistical software suite.
-Orange Data Mining
Orange Data Mining, a free and open-source data mining program, includes predictive analytics techniques.
These are only a few of the numerous predictive analytics solutions currently offered on the market. Make careful to take your company’s needs and budget into account when selecting a predictive analytics platform. You can advance your company with the appropriate predictive analytics tool.
Business intelligence software advances daily, using algorithms, artificial intelligence, and machine learning to comprehend our business decisions more thoroughly and predict future trends. Predictive analytics software is helpful in this situation since it helps us identify potential risks and expansion opportunities.
According to industry forecasts, the market for predictive analytics software will increase significantly over the next few years, rising from its current value to around $21.5 billion. This number might be hard to accept, but it would still amount to a considerable gain of almost four times the market value. As a result, we should consider this prediction carefully.
By utilizing already-existing data, predictive analytics solutions assist in identifying trends and best practices. To evaluate risk and provide fraud outlooks, marketing departments can use this program to identify new client segments by banking and insurance firms. Additionally, businesses in the manufacturing and retail sectors forecast changes in demand or the impact of potential process changes on their supply chains.
Check out the list below if you’re unfamiliar with predictive analytics solutions. Within its three segments, some of the most significant merchants are present.
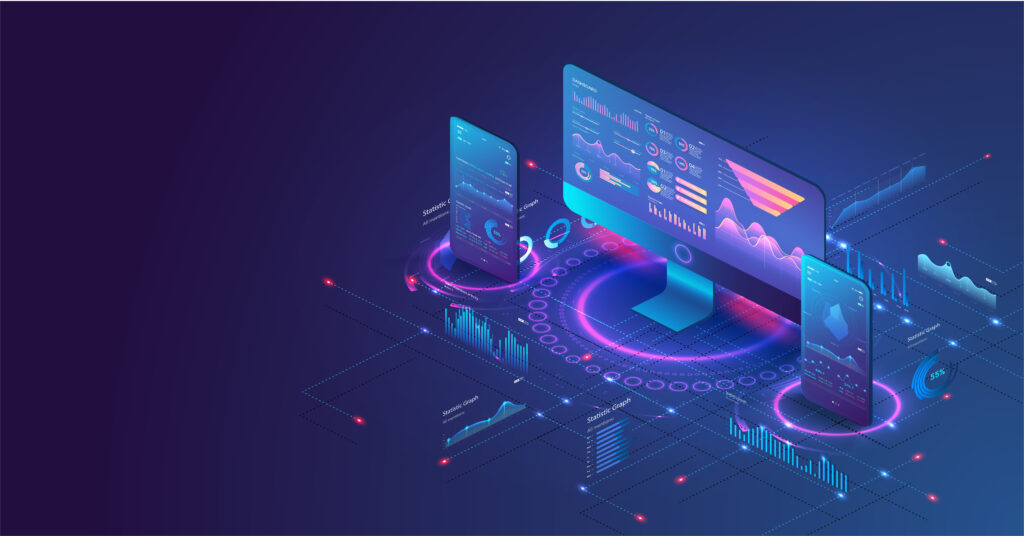
Predictive Marketing Software
-ZoomInfo
Businesses may use their marketing data to create highly individualized segments with the help of ZoomInfo’s data curation and mapping technologies. To build an effective database, all of this data is combined with your site activity data and the current client profiles. In essence, ZoomInfo produces corresponding client categories based on accounts and contacts that are most likely to convert after you tell it what you’re looking for.
ZoomInfo allows you to segment your target demographic and develop unique messaging for every customer journey. It is the perfect tool for account-based marketing campaigns because of this.
-Infer
Predictive modeling from Infer combines all your data sources to understand where a lead is in the sales funnel thoroughly. For their accounts, Infer increases conversion rates by three on average.
Infer keeps an eye on online resources and open data before creating predictive models using previously established key accounts and criteria. All of this information will be helpful for account-based marketing and sales prospecting teams to find prospects who are prepared to convert and shorten the entire sales cycle.
-Radius
Radius offers various data analytics services focusing on predictive B2B marketing. Several essential characteristics include:
- You can work with other companies to target specific consumer segments and share audiences through the Radius Customer Exchange (RCX), a marketplace.
- Thanks to Salesforce: Radius Connect, finding and acting on your most crucial data is simpler than ever. Using Salesforce, you can link conventional reports with real-time analytics provided by B2B platforms like AI Engage, Infinite Chindia, and Heap Analytics. You can quickly reach more competent judgments by delivering detailed predictive data to Salesforce so that your insights are accessible where your sales team is most productive!
Like Infer and EverString, Radius lets marketers exchange data and find new accounts within their databases. Another cloud-based system is Radius.
Industry-Specific Analytics
-Logility
A data analytics and predictive solution made exclusively for supply chain management is called Logility. Logility’s comparative analysis of existing data, based on a business intelligence platform, enables customers to create “what-if” models for more intelligent supply chain optimization.
A quick and easy way to perform a risk analysis, including the identification of risks and their repercussions, is provided by the Logility platform. Because Logility is a user-friendly, cloud-based platform, you can access your data from any place and keep a watch on your supply chain in real time.
-BOARD
By inputting new statistics into a few text areas, you can use the interactive dashboard to display specific information about your key performance indicators (KPIs) and analyze the outcomes of alternative hypotheses. Our “non-transactional” architecture makes this possible, which enables us to respond swiftly to shifting circumstances without frequently updating our models.
The OLAP cube, cloud database, flat files, and your ERP system are just a few data sources that THE BOARD development framework may access. Additionally, you can create custom applications based on your forecasts using the BOARD toolkit. THE BOARD is an excellent option for the banking, insurance, retail, manufacturing, and logistics sectors.
-Statistica Decisioning Platform
The Business Intelligence toolkit from Statistica comprises several products that work together and independently. You can use the Decisioning Platform, a predictive analytics tool, to create more effective and flexible business decisions. The software forecasts consumer and market behavior, enabling you to spot business opportunities by applying custom corporate and situational restrictions (such as state and local legislation) and seeing trends in your company data.
To create comprehensive prediction models, download and install other Statistica tools. Although any company can use this software, it has a history of generating fraud and risk models for the financial and insurance industries.

Analytics for the Data Scientist
-SAS Advanced Analytics
The company, which has been in business for 20 years and controls 33% of the predictive analytics industry, transitioned from code-based analytics divided among multiple divisions to visual, self-service editors that enable even beginners to perform advanced data analysis.
Major features include:
- Pictures, both real and imagined
- An automatically produced process map
- embeddable script
- Scheduled and automatic regulations
SAS might be a suitable choice if you’re seeking software that can quickly handle massive data sets while predicting movements. To begin, the business provides free trials and a tutorial-filled assistance center.
-RapidMiner Studio
For individuals who need to prepare and analyze data, RapidMiner Studio is an excellent tool because it makes it simple to implement unique business solutions. With its code-optional interface, you can automate reporting based on specific periods or have changes triggered by occurrences in your visualizations. Additionally, you may import your own data sets and export them to other programs thanks to the platform’s 60+ native integrations.
RapidMiner’s flexibility for data scientists can be increased via extensions (for instance, anomaly detection, text processing, and web mining), although they are not without cost. Although RapidMiner was created with data scientists in mind, it is simple to set up and use. You could even be able to begin using a limited-edition free version of the product from the website (though it would be restricted to one logical processor; customer support would not be available).
-IBM SPSS
Software for data modeling and statistical analysis is called SPSS (formerly Statistical Package for the Social Sciences). Structured and unstructured data are both supported by the program’s capability. This program may be downloaded in the cloud, on-premises, or through a hybrid deployment to satisfy security or mobility requirements.
SPSS offers a modeling dashboard that builds predictive models from your existing data. Premium unstructured data support includes linguistic and natural language processing, enabling you to include social media and other text-based sources in your models. Additionally, the application has entity analytics, which can be utilized for customer management and security planning to de-duplicate your data and detect linkages between entities.
-SAP HANA
An in-memory database and application service called SAP HANA also provides cloud-based options. With SAP, you can create predictive models from any linked application using any data set you provide. This tool, in particular, uses additional connections for “external large data” sets and straightforward visual workflows to cut down on the time it takes to construct your models.
The future of analytics is palettes. Based on users’ prior actions, they are a group of algorithms that “predict” how they would respond to goods and services. The software analyzes text and social network data to identify consumer trends and the products they may be interested in based on past behavior.
You can write your queries using the programming language you already know because the program is R code compatible. When enough internal data has been gathered, predictive models will automatically give fresh perspectives.
As you create your predictive analytics plan, keep your current systems in mind, such as supply chain management, CRM, ERP, marketing, and human resources. While some of the provided things are helpful for regular business users, others may annoy less-experienced analysts who feel obligated to rely on database administrators to connect sources and change reporting criteria.

Why Predictive Analytics Matters
-Rise of Big Data
Predictive analytics is often brought up while discussing extensive data. Big data is a dataset that is too large and complex for standard data-processing software to handle effectively. Massive data is another term for dark data. Predictive analytics and other business intelligence technologies enable analysts, managers, and executives to make informed decisions based on their goods, services, clients, or anything else they choose!
-Increasing Competition
Businesses try to gain an advantage in crowded markets by learning more about their competing market. Data-driven predictive models can help firms find novel solutions to persistent problems.
Likewise, it might be more challenging for equipment manufacturers to create new gear. To increase the value to the client, product developers can include predictive capabilities in already-available solutions. Predictive maintenance is also known as predictive analytics.
Predictive analytics increases conversions and enables businesses to forecast demand more precisely. For instance, electricity companies can plan their power plants based on these projections to anticipate client demand. This leads to a significantly more effective resource planning process by forecasting the demand for electricity on the electrical grid.
-Cutting-Edge Technologies for Big Data and Machine Learning
Businesses apply algorithms to big data sets using platforms like Hadoop and Spark to extract value from enormous amounts of data. The data sources could be transactional databases, equipment log files, pictures, videos, audio, sensors, or other information. Innovation frequently comes from combining facts from various sources.
With so much data, algorithms are now required to glean insights and patterns. Machine learning techniques are used to find patterns in data and develop models that project future results. Some machine learning methods include neural networks, support vector machines, decision trees, and linear and nonlinear regression.
-Predictive Analytics Examples
Teams across various industries, including banking, healthcare, pharmaceuticals, and automobile production, can benefit significantly from predictive analytics.
- It won’t be long before we see more electric vehicles in our daily lives as their popularity grows. The auto industry will undergo a revolution.
- Businesses employ predictive analytics to examine historical driver data to create new autonomous car technology. This benefits the drivers and improves road safety.
- Aerospace – Ensuring the health of aircraft engines
- An engine manufacturer developed a real-time analytics tool to forecast subsystem performance for oil, fuel, liftoff, mechanical health, and controls to increase aircraft uptime and save maintenance costs.
- Energy Production: Predicting demand and price
- Apps that produce accurate forecasts use models that consider plant availability, historical trends, yearly cycles, and weather.
- Constructing credit risk models for banks.
- Financial organizations calculate credit risk using machine learning and quantitative methods.
- Industrial automation can prevent expensive mishaps and production halts by foreseeing equipment faults.
- A plastic and thin film manufacturer uses a health monitoring and predictive maintenance program to lower downtime, minimize waste, and save 50,000 Euros per month.
- detection of asthma and chronic obstructive pulmonary disease using pattern-detection algorithms
- To help patients manage their asthma and COPD, an asthma management gadget records their breathing and offers feedback via a smartphone app.

How Predictive Analytics Works
Predictive analytics is the application of data analytics to create predictions based on historical data. This approach builds a predictive model to forecast future events using data, analysis, statistics, and machine learning methods.
Using a mathematical or machine learning technique to provide a quantitative future prediction is called “predictive analytics.” Supervised machine learning techniques are typically used to estimate a probability (How likely is it that this customer will default on a loan?) or predict a future value (How long may this equipment function before requiring maintenance?).
Predictive analytics uses data to cut expenses, save time, and reduce waste. This process transforms diverse, frequently large data sets into models that can produce precise, actionable results like reduced material waste, decreased inventory levels, and manufactured goods that adhere to specifications. As a result, the business meets its initial objectives.
Predictive Analytics Workflow
Predictive models for predicting the weather are commonplace. Energy load forecasting, used to forecast energy consumption, pertains to an essential industry use of these models. To manage loads in the electric grid, energy producers, grid operators, and dealers in this situation require precise energy load estimates. There are a lot of data sources that can be combined with predictive analytics to give grid operators helpful information.

Where is predictive analytics used?
Predictive analytics can be used in a variety of applications and industries with the help of these new skills. Here are some illustrations. To predict stock prices and other financial indicators, financial institutions rely on it. On the other side, banks, mortgage lenders, and credit card businesses seek to spot fraudulent transactions, give their best clients the most significant discounts, and market cutting-edge financial products to new clients. In each of these cases, predictive analytics has already proven valuable.
Similar algorithms are used by other consumer-facing industries, including retail and telecommunications, to manage customer interactions. Churn analysis is a tool to determine whether consumers are potentially dissatisfied or likely to switch carriers or services. These businesses can take measures to raise client satisfaction and keep them from leaving if they are aware of this information in advance.
It’s not always simple for airlines to forecast how many seats will be occupied on a given aircraft. Think about all the times you’ve tried to book a flight, and there are only a few choices because the airline misestimated the demand for that route. The same principle applies to logistics firms using predictive analytics to attempt and improve their supply chains. That method also contains a great deal of uncertainty.
How to get started? Benefits of predictive analytics
How can we use predictive analytics effectively, bearing all of this in mind? I like to offer three straightforward scenarios because they may be applied in several industries and already have the necessary data. The actual methods are likewise pretty simple. Finally, you may just implement your plan and assess the results.
-Time series analysis
A time series is any data that documents how values change over time. Time series data can include daily sales, weekly invoicing, monthly expenses, or your annual budget. But other changes over time, such as the temperature at which some equipment operates or the number of website visitors, could also be regarded as time series data.
This can benefit your company in some ways. Just consider what you could do if you had the ability to forecast based on the facts you already had. What will our monthly revenue be? What is the probable average invoice value for the upcoming year? Maybe the temperature at which this equipment operates is higher than usual—possibly dangerously so? These estimates could be advantageous to almost every organization in some way.
Although there are sophisticated algorithms for movement prediction, moving averages are a more straightforward option. Although these can be completed in Excel, they are often employed in the stock market and commodity analysis. During their undergraduate years, many statistics, engineering, and marketing students study algorithms like ARIMA (AutoRegressive Integrated Moving Average), which are eventually used on business intelligence platforms. Some of these algorithms even have the ability to alter forecasts for seasonal variations.
You may conduct informed, fruitful business talks about present patterns and potential futures with even the most basic time-series analysis.
-Cluster analysis
We know that getting new clients is never simple because they constantly worry about missing out. In the preceding case, young people purchased things with more features, whereas elderly people might have paid more for superior quality. Divisions on the page might be seen if customer age and spending were mapped. Consumers who are younger and spend less money tend to be older and spend more, with middle-aged customers possibly being the most expensive of all. Additionally, a few anomalies dispersed around the periphery do not fit into a group.
Your business may be able to make a ton of money from this. These trends are found using cluster analysis in several different ways. Then you may use these groupings for targeted marketing or to help you create things that will sell well. Even those outliers may be examined to determine why they don’t seem to fit together. Their spending may be extraordinarily high or low due to managerial fraud or ineptitude. These details are relevant to salespeople.
-Recommender systems
This scenario is one that we’ve all seen before. At its best, an analytical engine may persuade us to make a new purchase or take on a new behavior without even being aware. Of course, the most straightforward argument is that customers who bought X were likewise likely to buy Y. They also watched A and B. Therefore, we suggest trying B as an alternative.
These systems can be built with specific data, such as the customer’s ID and the product ID they choose. Include details like dates of certain occurrences if you want to be more explicit, for example. But starting small is simple.
To maintain these systems functioning properly, there are a few difficulties that we must be careful to avoid. The first is the application of patterns and guidelines without a lot of supporting data. For instance, it is insufficient of a recommendation to base our choices on if only one consumer purchased items A and B together. To prevent recommending products to our consumers that they already own, we also need to be aware of what they have previously purchased from us. It would make us appear dumb to do so.
Not all recommendations are based on viewership or revenue. They can also be used for navigation apps and equipment maintenance.
-A final prediction
These three quick wins can be ideal for getting started with predictive analytics if you have never done it before. Nevertheless, predictive analytics will play a part in your company’s future, regardless of your business interests or the challenges it is currently facing.
Conclusion
Although the topic of predictive analytics is complicated, its advantages are obvious. Businesses can future-proof their operations by employing predictive analytics technologies to help them make better decisions based on historical data. We have included the top ten predictive analytics tools in this blog article, organized by category. Please contact us if you want to learn more about predictive analytics or start utilizing these technologies in your company.